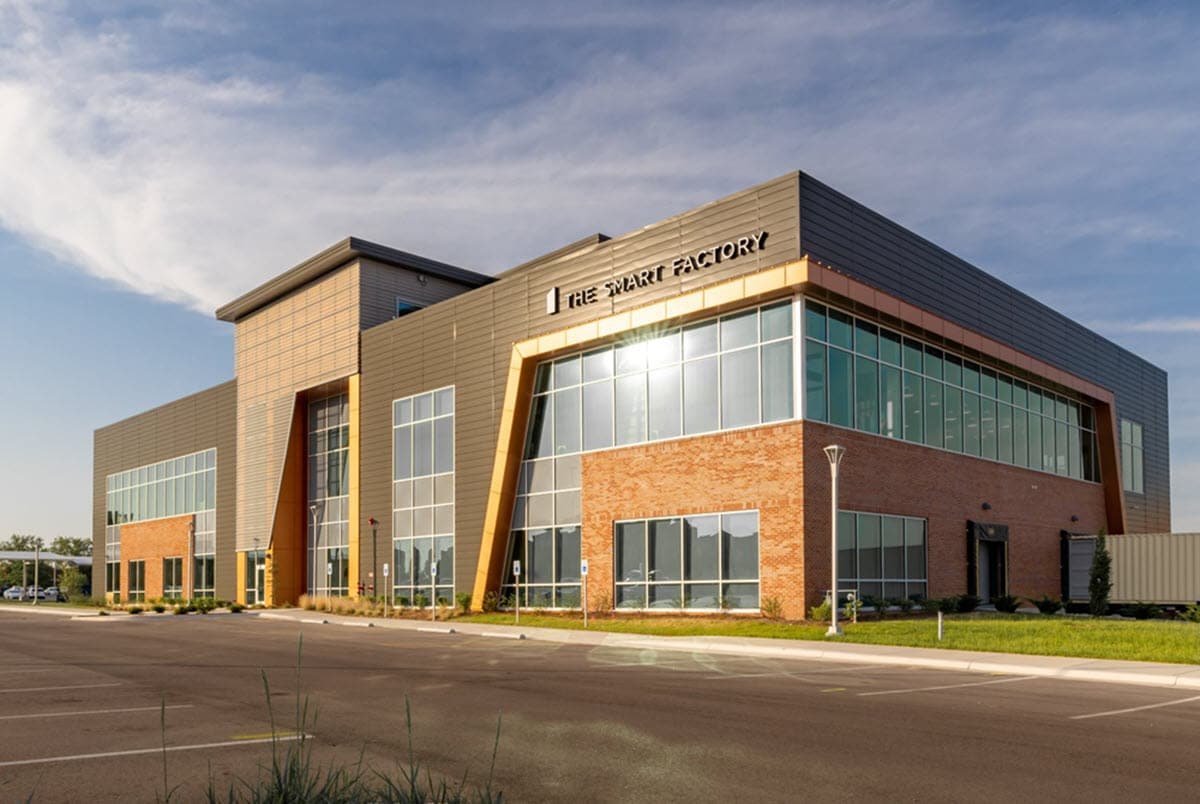
Deloitte.
Smart Factory Product
The Smart Factory Product is an Industrial IoT product hosted on AWS that delivers improved manufacturing operations through real-time analytics and data-backed insights. This is made possible by enabling managed services and unifying the current IT & OT solution architecture through homegrown modules and extensibility. The product aims to deliver a sustainable factory of tomorrow while augmenting, rather than replacing, todays workforce.
Impact & Role
I have operated as the product manager and functional lead for the project since its conception. Our team has steadily grown from a strength of 10 to over 100 practitioners. The product build followed an Agile software development lifecycle process that was made possible by the Scrum methodology. My role included five primary responsibilities :
Running design thinkings sessions with stakeholders to define the intent of the product
Creating functional prototypes and iterating with designers to develop high-fidelity screens that showcased user scenarios
Defining algorithms and mathematical formulae that translated machine data into manufacturing insights
Drafting detailed user stories and presiding over scrum calls to ensure software development is aligned with the functional vision
Running quality assurance assessments post-development of each user story to ensure error-free deployments to the Production environment
Design Journey
Once the key modules were identified, we ran design thinking sessions with subject matter experts to identify and map the information they deemed to be the most critical and insightful to help operators on the shop floor make data-backed decisions
The team was tasked with curating a list of Smart Factory applications to deliver a minimal viable product (MVP) that would be deployed at our Smart Factory at Wichita (SF@W) as an accelerator for clients to realize the power of our product. This was treated as a proof of concept before further investment from the firm. The project details below relate to the aforementioned MVP. Any references to the ongoing market-relevant product build have been blurred or consciously not shared to protect the firm’s intellectual property.
Our team identified a need to design a user experience that was native to the manufacturing industry but one that also pulled from Deloitte’s considerable experience in building custom applications. For this product build, our team was tasked with meeting with industry experts to identify the most critical information that each product module should source from programable logic controllers and feed to the screen. Our design discussions also included debates on how certain key performance metrics should be calculated based on the different manufacturing archetypes, namely continuous, batch, and discrete manufacturing.
1
2
To kickstart the product build we ran a market analysis to identify the most critical use cases we want to build as a next-gen IIoT platform. Our team also sifted through smart factory custom application development projects Deloitte has delivered over the past 10 years to identify best practices and key learnings to bring the best to this comprehensive cross-industry product.
Our team went about categorizing application types and prioritizing builds by the level of effort and market demand to deliver a minimal viable product (MVP). (Blurred to protect firm IP)
For each module, we developed functional prototypes that stripped the screen down to the bare-bones functionality by defining data tables, user input fields, calculated data fields, and action buttons
The functionality was iterated over with SMEs to identify improvement opportunities based on industry relevance and standards
3
After locking down functionality, we outlined where each functional element would be positioned on the screens based on the hierarchy of the information
4
We worked closely with our design team to translate the functional prototypes (step 3) to low-fidelity clickable prototypes on Figma. Leadership reviews were conducted once again, and the low-fidelity screens allowed decision-makers to make objective calls on the workflow of each screen.
5
Delivering high-fidelity prototypes was the last step of the design process. The team applied UI elements from our master component library. Our team provided ‘scenarios’ that depicted use cases for the module, and clickable prototypes were stitched together to walk stakeholders through the enter user journey for the screens. After final feedback was incorporated, the screens were handed off to the front-end developers
6
Functional Logic Base
As an Agile product build, an attempt was made to break every functional module into bite-sized user stores to make the stories easy to digest, maintain a high development velocity, and allow the QA team to test without ambiguity. Shown below are extracts of algorithms, mathematical formulae, and sample data scenarios that were paired with user stories to guide the developers to code the logic
1
Calculations were visualized for developers to get a clear understanding of variables involved, types of interaction, and business scenarios that triggered calculations (Draft sample shared to safeguard firm’s IP)
2
Decision trees were created where appropriate to represent various business scenarios. Such decision trees were presented and debated over in daily scrum and technical solutions calls. They proved as vital hand-off documents between the functional team and developers to avoid misinterpretation of complex business rules detailed in user stories
3
Show alongside is an example of typical mathematical formulae the functional team designed. These technical snippets provided the developers with a detailed breakdown of the key variables and formulae they had to bake into the logic engine of the product. A general overview included the formulae themselves, units of measure, expected data sources, and expected refresh rate for optimal performance. (Draft sample shared to safeguard firm’s IP)
4
Developers and quality assurance were provided with a comprehensive list of error messages with sample data that reflected each error scenario. This helped reduce ambiguity when translating error rules and interpreting the data they stemmed from.
Shown here is a data upload sample. The first table displays a sample of a perfect, error-free upload data table that the system may expect from the user based on the specific data type in each column. The tables that follow each visualize a rule that has been broken, by highlighting unacceptable data in red, with the error message that should be displayed
Smart Factory Minimal Viable Product
1
2
The landing page was developed for a managerial persona to get an overview of the health of all their manufacturing sites. Pins denote the location of each plant and their color denotes its real-time health based on overall equipment effectiveness at the plant. The user can click on any pin to view details of a specific factory
The factory health module provides a real-time view of the factory’s health based on throughput and work-in-progress. The ‘health’ is measured by referencing configured values for thresholds
3
4
The overall equipment effectiveness module provides the real-time OEE values at the plant, area, zone, and line level in an aggregated manner. Financial losses are also reported when the plant is performing under desired effectiveness
The constraint management module reports the current open equipment constraints with their location and duration metrics. The user can double-click to understand the underlying fault and work order status to remedy the constraint.
5
6
The standard operating conditions module provides a real-time view of the most at-risk equipment categories based on their operating conditions relative to performance per the engineered design
The configuration module ensures the application can be tailored to the needs of myriad discrete manufacturing clients. Seen below is a sample of the general configuration screen where the user is defining their weekly schedule
The Smart Factory Product will enable next-gen factory operations at Deloitte’s test bed in Wichita State University and numerous clients that purchase the product license
Disclaimer: All data shared on this page is considered the property of Deloitte Consulting LLP. The author does not claim rights to any information and has only provided the same for an academic evaluation